Blog
Unlocking AI’s potential in anti-corruption: Hype vs. reality
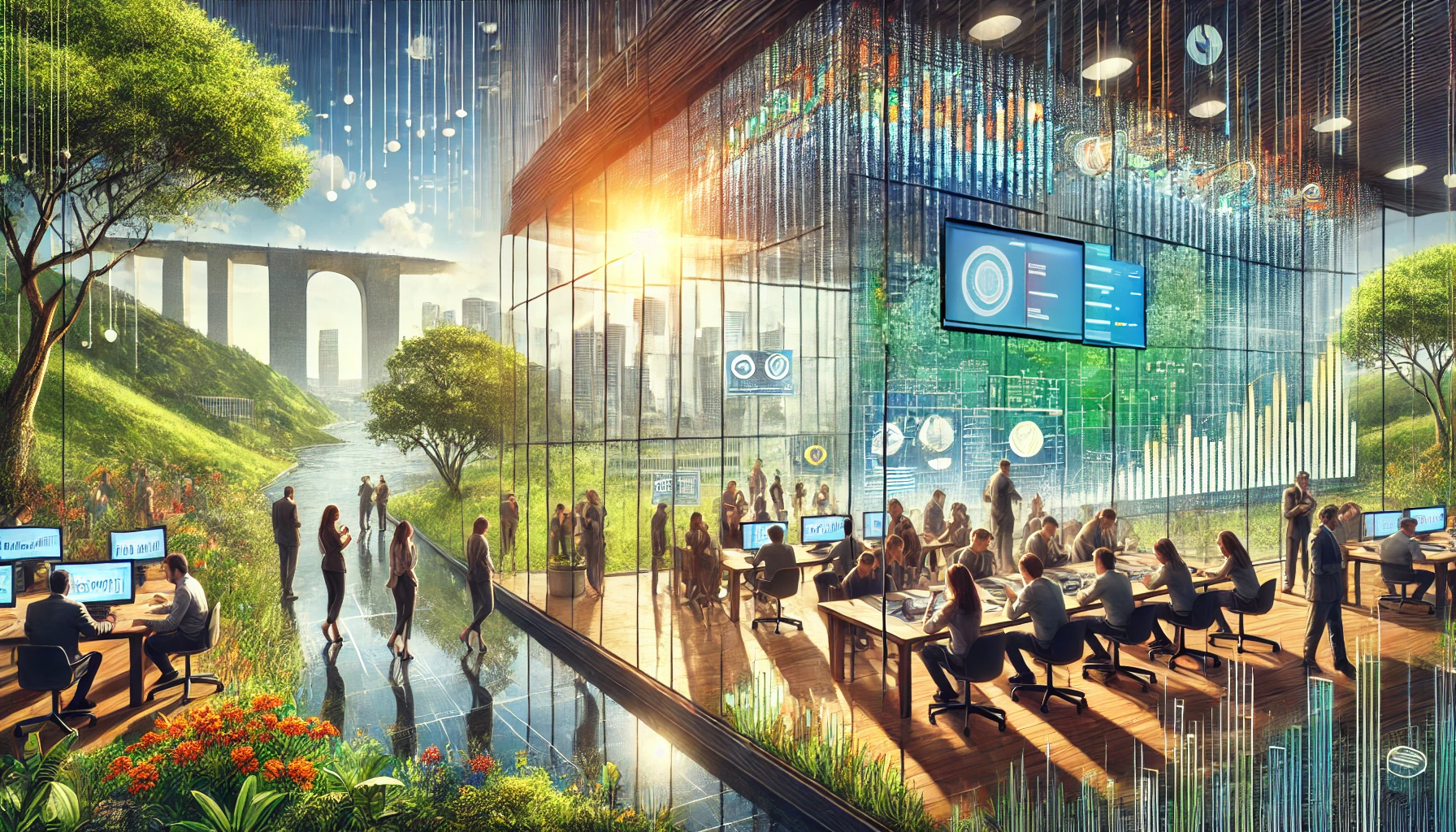
A U4 panel discussion with artificial intelligence (AI) experts reveals many opportunities – and challenges – for using AI in anti-corruption efforts. The event confirms that, while there is much potential, the successful deployment of AI needs human oversight to improve data quality and ensure long-term sustainability. By highlighting some of the real-world examples, we assess whether AI can empower anti-corruption stakeholders.
Can AI support anti-corruption work?
As the following examples highlight, AI anti-corruption tools (AI-ACTs) offer innovative ways to support anti-corruption work. AI-ACTs can analyse vast amounts of data, flag irregularities, and improve governance oversight. The technology can detect abuse in public procurement, and monitor large-scale infrastructure projects.
Protecting public resources
In Brazil, the Alice bot helps auditors analyse tenders, bid submissions, and public contracts. The technology highlights potential issues such as embezzlement and anti-competitive practices, alerting assessment teams before final procurement decisions are made. A recent study shows that Alice significantly improved the government’s ability to identify fraudulent claims. This improvement reduced financial losses in audited cases by 30% and strengthened safeguards for public funds.
Detecting suspicious language
Large language models (LLMs) have been effective in detecting suspicious language in email exchanges – such as the functionality used by the European Anti-Fraud Office (OLAF). Machine learning can also help conduct risk assessments and detect anomalies. For instance, the Datacros tool, adopted by public authorities in Romania, France, and Lithuania, alerts risks of collusion, corruption, and money laundering.
Finding irregularities in public expenditure
In Brazil the bot Rosie has been used to check parliamentary expenditure and detect irregularities. Unfortunately, while the technology worked, the evidence it provided was often insufficient for prosecutors to open a legal case. After the initial excitement, attention waned, and the bot is no longer active.
Detecting corruption in roadworks and mining
In the Democratic Republic of the Congo, AI combined with satellite imagery has been used to detect corruption in road construction and mining projects. The technology analysed discrepancies between satellite visual evidence and reported progress. However, recent information on this initiative is unavailable. Long-term success depends on the continuous use of the AI technology and the preservation of working partnerships.
Preventing online data fraud
Data quality is another important consideration. MasterCard successfully uses generative AI to detect compromised card information on the dark web. The technology scans millions of merchant transactions to cross-reference with internal databases to detect and prevent fraudulent transactions. However, inaccurate, outdated, or biased data can lead to incorrect or inconsistent results. This can undermine fraud detection by either missing patterns or over-reporting suspicious activity. Also, in some countries (such as Norway) public services are highly digital, but in many other regions, the necessary data infrastructure does not exist in digital form.
Predicting corruption in the civil service
Data quality and data availability are equally important issues when applying AI to other areas, such as predicting corruption. AI can be used to predict patterns of abuse by analysing behavioural data, communication patterns, or online activity for early warning signs.
Brazil’s Mara uses machine learning algorithms to assess data on civil servants who have been previously caught and punished for corruption. To improve oversight and prevention, Mara identifies patterns such as career history, involvement in specific projects, and political affiliations. The AI ranks individuals based on their likelihood of engaging in corrupt activities.
However, Mara has faced criticism for reinforcing biases, as it is trained solely on individuals who have been caught and punished. This may exclude undetected corrupt behaviour and disproportionately focus on civil servants from agencies with stronger internal oversight. Also, relying too heavily on Mara’s rankings risks overlooking broader systemic corruption and may foster a false sense of security.
Identifying benefit fraud
The Dutch tax authorities used an AI-driven tool to identify benefit fraud. This case highlights the dangers of unregulated AI in governance. The AI identified ‘dual nationality’ and ‘low income’ as indicators of high risk. However, without sufficient oversight, categorising individuals based on these factors resulted in harm to vulnerable groups. The tool was eventually withdrawn.
Uncovering irregular transactions
China’s Zero Trust programme was designed to detect irregular financial movements by civil servants, and calculate the likelihood of corruption. It identified more than 8,700 officials (from the government’s payroll of more than 60 million people) engaging in questionable transactions.
While effective, the programme raised significant concerns about privacy and surveillance. It was ultimately discontinued to avoid large-scale resistance from bureaucrats, including those in powerful positions.
Enhancing project design
One promising area of research is the application of AI in agent-based modelling (ABM). In ABM, AI simulates the interactions of agents to analyse social dynamics, including behaviours, triggers, and drivers. This new approach involves constructing ‘digital twins’ of communities, enabling the observation of how virtual agents influence each other in their environment. This has the potential to enhance project design through participatory modelling. It could also test theories of change in anti-corruption programming and support anti-corruption strategies.
Human oversight of AI remains essential
Many AI-ACTs are relatively new, and long-term research on their impact remains limited. While the examples highlight AI’s potential, they also show its limitations. The weaponisation of anti-corruption efforts and the rise of populist regimes underscore the need to address broader ethical and societal implications, such as surveillance concerns and the potential misuse of AI. Human oversight remains essential to ensure that AI processes are based on accurate interpretations and actions. By examining the successes – but also learning from the challenges – we can unlock the potential of AI to enhance anti-corruption efforts.
Our AI expert panellists have a number of recommendations:
Integrate AI into existing anti-corruption frameworks: AI-ACTs have been most successful when they are embedded into existing processes, and complement existing anti-corruption efforts, rather than replacing them entirely.
Address risks and biases: The public sector needs to understand how AI-ACTs work and find ways to mitigate risks. This can be done by implementing proper checks and balances and dedicating adequate resources, for instance partnering with civil society organisations to check algorithms and ensure accountability and transparency in the use of technology. Software can also be used to mitigate bias.
Incorporate diverse perspectives in AI design and implementation: It is crucial to have teams of people with different views and perspectives. A diversity of voices should be included from the early stages of AI development. Different areas of expertise (eg, sociologists, economists) should be included alongside representation of diverse gender and intersectional identities and experiences. If these perspectives are not already represented, they should be actively sought during feedback and testing stages.
Supported AI with a sustainable business plan: Many of the AI initiatives of the last decade have had short lifespans. This has been due to limitations related to data quality and the biases present in the development and use of these tools. The failure of AI has also been due to resistance to change, breakdown in coordination among stakeholders, or the lack of a sustainable business plan. The successful use of AI technology requires sustained effort and support from multiple actors. The technology needs a solid ‘business plan’ to ensure sustainable, long-term collective action.
If you are interested in exploring these opportunities further, please get in touch with the authors.
Acknowledgements:
We would like to thank the AI experts we consulted and who attended our panel discussion in June 2024: Fernanda Odilla, researcher on anti-corruption and AI at the University of Bologna; Carolina Gerli, PhD Candidate at the University of Bologna; and Giovanni Leoni, Global Head of AI Governance Advisory at Credo AI.
Disclaimer
All views in this text are the author(s)’, and may differ from the U4 partner agencies’ policies.
This work is licenced under a Creative Commons Attribution-NonCommercial-NoDerivatives 4.0 International licence (CC BY-NC-ND 4.0)